At Blueprint Technologies, one of our key practice areas is data science and analytics. However, we still find that many do not understand the basics of data science, nor do they accurately estimate the benefits to be gained from getting the very most from their company’s data. We interviewed our Solutions Architect and Data Science expert, Manish Shukla, to help answer some of the most asked questions.
When we talk about data science at Blueprint, what do we mean by that exactly?
MS: Data science is the process of collecting raw data, extracting information out of it and gaining insights from it. That’s it in a nutshell, and there are different mathematical and statistical techniques applied on data to get those insights.
What is Blueprint’s perspective on data science?
MS: Blueprint looks at everything through the lens of digital transformation. One of the pillars of digital transformation is the constant drive to make companies or clients more data-driven. The best way to make your organization more data-driven is to efficiently consolidate different sorts of data to derive insights and analytics from it to drive more revenue and diversify your revenue proposition. Data science lets you predict for the future based on historical data so that you can, no matter the industry, answer the big questions. How do you handle your inventory? How do you optimize your supply chain? Are there key market segments you’re not reaching?
Exciting correlations and new possibilities for innovation and improvement begin to appear as more and more teams make impactful decisions based on data science. At this point your data science practice will be an integral part of your organization and strategy.
Manish Shulka, solutions architect and data science expert
What are some of the major components of a data science practice?
MS: A major part of establishing a data science practice is defining key measures, metrics and key performance indicators You can build mathematical models, deep learning or artificial intelligence, but how do you measure success? It’s crucial to do that definition-based work early and revisit it often, and it’s also key to allow for non-financial success metrics to ensure you consider all success factors.
Data science is a very complicated power. It’s very R&D focused space, and it’s not your typical IT space where you have a fixed or expected output. Often you don’t know what you are looking for. You’ll need to do some R&D and budget around it, but organizations often find that challenging, particularly in the time of COVID. A lot of companies are freezing spending because they feel like, “I have no revenue. Why should I invest in data science?” The truth is that this is a perfect time to invest in data science and other digital transformation strategies, if for no other reason than your competitors probably aren’t.
You also need to develop the strategies around how you monetize your data and your data science practice, because no matter how many non-financial factors data science can influence, you’re always going to need to justify that expense with revenue.
Another factor to consider is how you will assemble the right talent and technology to run your data science practice. How do you find the right talent? The right infrastructure? The right set of technologies and platforms? Because data science comes with the challenge of a wide set of skill sets in the talent pool. There are literally a hundred different varieties of data scientists who are proficient in varied languages, technologies and platforms, all using different open source packages and enterprise tools, and IT organizations often find it very challenging to support that. Many companies aren’t built to handle the ever-changing pattern of data science.
So, you have to have the right combination of talent and infrastructure but do not disregard data security. You must have the right set of security systems and protocols in place due to the high likelihood that you’ll be handling personal identifiable information (PII).
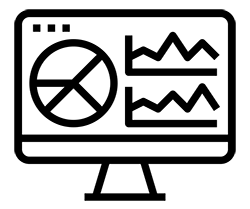
What are the signs that it’s time to build my organization’s data science capability?
MS: When you have questions about your business that you can’t answer, that’s a big sign. In a retail setting, the head of sales may observe a high level of customer churn and feel that is impacting the bottom line. But how can she go about proving and quantifying her assertion with real data?
The head of sales could then assign resources to building a descriptive dashboard, a true 360° view of the data. With a descriptive dashboard she would have access to information across all aspects of the business, including the ability to segment data regionally, by product mix or demographically. Then she can begin the investigation needed to get to the bottom of her churn problem.
Once you start walking down that road, you will learn you are not capturing some key data in your digital processes. Then you will need to go back to your data pipeline process and begin to capture that data.
While you’re answering your immediate questions, you’ll find that your data science practice is producing interesting insights from all over the business. Exciting correlations and new possibilities for innovation and improvement begin to appear as more and more teams make impactful decisions based on data science. At this point your data science practice will be an integral part of your organization and strategy.
What are some of the common steps that organizations go through when they build up their data science capabilities?
MS: The first step is usually an audit of the data they already have in-house, along with the major strategic goals and KPIs of the business. Then companies usually enter a planning phase, where they select appropriate initiatives and goals and agree to timelines and assemble teams to do the work. One major way many companies struggle to establish a data science practice is struggling to identify how to find ROI quickly. Many companies will start the process by hiring a data science team and then lose momentum when they fail to see high value quickly. But by skipping the first several steps of the process, they’ve virtually guaranteed sub-optimal results.
What are some of the expected benefits of a strong data science capability?
MS: The benefits come down to prescriptive and predictive data and the decisions data drives. Predictive data gives you the insight needed to make data-driven decisions and plans, and prescriptive data provides recommendations based on that insight.
A hospitality company might wonder, “How do I get more customers? How do I sell more products? More service offerings? How do I grow my business?” If they have a mature data science capability, they can better answer those questions and accelerate that growth.
A strong data science practice helps your data engineering, application development and mobile teams to launch better products. Data that was stored but not fully utilized may start returning interesting insights. A fully developed data science practice will enable companies to continue to drive further into technical solutions and decision making that slower competitors cannot duplicate.
Want to read The basics of data science: part 2? Click here