Have you read The basics of data science: Part 1? Click here
At Blueprint, one of our key practice areas is data science and analytics. However, we still find that many do not understand the basics of data science, nor do they accurately estimate the benefits to be gained from getting the most from their company’s data. We interviewed our Solutions Architect and Data Science expert, Manish Shukla, to help answer some of the most asked questions.
What are the opportunities companies miss out on if they don’t develop their data science practice?
MS: If you do not invest in and maximize data science, you can never be a data-driven organization. That is a very powerful, but accurate statement. All organizations must make decisions based on the best, most relevant and accurate data, but many misunderstand resource allocation. Data lakes and BI teams don’t make an organization data-driven. Without data science, all you have is historical data in an “as-it-is” state, with no ability to use it to predict and plan. That ability comes through investment in data science. Without it, companies will always trail competitors who can make data-driven decisions.
A thriving data science practice also grounds the entire organization in one rock-solid point of truth for data. Data science gives you more options, tools and overall firepower, so not investing in data science is truly a losing proposition for anyone.
What are some of the challenges to starting that process they might not be aware of?
MS: Building a data science pipeline is not an easy process, but with some forethought many challenges can be avoided. The first key is to take the time to plan and consider your resources. Often a data scientist will want to investigate something, so they will get access to some data, analyze it and circulate some derived intelligence around the company. As more people want more, deeper analysis, they run into issues because the process is not scalable. It really is crucial to consider both infrastructure and end goals when developing a data science practice.
Team makeup is another key consideration that can help avoid challenges along the way. For example, there are generally three types of data scientists. Some have a strong background in software engineering, while others have a completely mathematical or statistical background. If the team is not properly constructed based on skillsets, they may run into difficulties when the process requires advanced coding, for example. It is vital that both data science and engineering teams be built in a balanced fashion so they can readily handle changing circumstances and demands.
Are there particular industries that benefit the most from a strong data science practice?
MS: It would be easier to name industries that wouldn’t benefit, but really any industry that relies on consumer demand would be well served to fully embrace data science. Whether it’s telecommunications, retail, finance, consumer packaged goods, the potential is massive. All those industries rely on and thrive when driven by consumer data. The use of this data to drive crucial decisions is the reason we invest in data science.
What is an example of a cool or impactful thing you have seen come from data science?
MS: I can think of a couple from my time working with Coca-Cola. The time I joined was an interesting time in Coke history, where people were worrying about the side effects of aspartame and becoming more health conscious in general. The soda business was certainly feeling those effects, and had been shrinking for some time, especially in the U.S.
I joined to help improve Coke’s data science practice and maximize its impact. Coke has an interesting challenge when it comes to customer data. Since Coke’s products are purchased mainly through grocery and convenience stores, it can be difficult to know enough about individual customers to effectively market to them, even at a regional level. Supermarkets and convenience stores share sales information with Coke, but they do not have the ability to share data on the customers doing the purchasing.
One way we began to collect data at the customer level is with the introduction of the Coca-Cola Freestyle machine. If you’re not familiar with it, the Freestyle is a soda fountain that allows customers to choose from any combination of 165 flavors of drinks, mixing them any way they like. The Freestyle is all about collecting customer preference data, and it has been a huge success. I included customer preference from the 20 million customers using the Freestyle machines as one of 30 different data sources that populated our data lake. Using the correlations we were able to determine through our work, we helped drive the addition of two new soda brands to the company’s portfolio, as well as determine the optimal regions to launch the new products.
What was the other one?
MS: It was around supply chain and inventory management. Coke had long had access to advanced demographic, financial and sales information, but the source of much of the data was external, so it wasn’t always exactly what we needed or in the format we would’ve liked. It was also a significant cost center. Once we had the data flowing into the data lake, though, we were able to eliminate that reliance on third-party data vendors and perform much more efficient, impactful data science. We were able to go beyond the basic daily sales numbers and provide reasons behind the performance, as well as recommendations to improve it. We derived recommendations in a number of ways, but one of the most interesting was by using natural language processing (NLP) to mine social media data to find interesting correlations. The impact was immediate and profound. Coke began to change many of their fulfilment methods and tactics to select, optimize and restock their products. This really brought the value of data science into focus, and soon Coke began partnering with supermarkets to add certain sensors to capture pictures and video, further expanding their available universe of customer data.
These are the kinds of advancements and capabilities that a strong data science practice can bring to your organization.
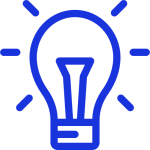
Blueprint Technologies is here to help you establish or improve your data science and analytics function and unlock the true power and value of your data.