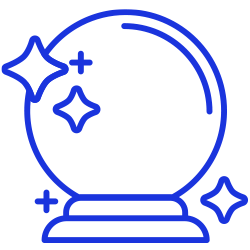
As technology changes how customers shop, dine and even commute, restaurant and retail companies need to establish a modern inventory management system to remain competitive – but to do that, they need to better understand demand.
Technology that utilizes data to optimize supply chains has made balancing supply and demand much easier, but entrenched organizations with multiple sales and technology solutions often find themselves in a bewildering maze of siloed data systems. The process is made much easier with a technology partner that understands supply chains and recognizes the value of optimization through data.
Customer demand drives future inventory needs, so any effort to forecast future inventory must begin there. The better customer demand is understood, the more accurately data models can ensure optimal stock levels and help determine when stock should be replenished.
Of course, no demand prediction model is 100% accurate, but there are methods that have been established over time and are still foundationally sound. This traditional process of demand forecasting involves five methods which work in concert to provide projections meant to be as accurate as possible given the data at hand. As industries advance and customer preferences shift, though, these methods must continually be modernized and improved to ensure they remain useful.
Passive demand prediction
Passive demand prediction is the simplest method and involves gathering sales and inventory data from previous years and assuming this year will be similar. For example, a restaurant may base their inventory and staffing levels for Cinco de Mayo, one of the busiest and most crucial days of the year for restaurants and bars, on their sales data from the last two years. Using this as the only method of demand prediction is easy, but also risky. Factors like day of the week, current weather forecasts and local events happening at the same time can impact sales widely from year to year, leaving companies unprepared to meet real-time customer demand.
Companies that aren’t taking these other factors into consideration are stuck in the past. Now is the time for restaurants and retailers to start using the full universe of data available to them or they will keep leaving money on the table.
Active demand prediction
Active demand prediction relies heavily on market research. Companies utilize in-house or third-party information to discover expansion and growth opportunities and utilize them to predict future customer demand. For example, a staffing agency could be informed by their market research department that Meta is planning to open a location in Austin, TX. This information would then trigger analysts to gather further market data for that location to inform a marketing plan to land that business. The drawback to this method is that it relies on skilled humans to wade through enormous volumes of data to pick just the right information to drive business value. A more modernized approach involves supplementing the work of analysts with video analytics systems, AI/ML and robotic process automation (RPA) to more efficiently sift through all available information to quickly identify promising opportunities, while allowing analysts to focus on the most high-value scenarios.
Short-term demand prediction
Short term demand prediction focuses on customer demand over the near term, usually the next three to twelve months. Traditionally, these forecasts are based on the historical knowledge of management regarding customer preferences and large events. As the pace of change quickens, this method has grown less effective. For example, an ice delivery company with many small towns in its delivery area needs to know and plan for any event which may affect demand in its area. In the fall, high school football games and tournaments do just that, as tailgaters spike demand. But what if there is an unforeseen global event, natural disaster or new trend? Relying on tacit institutional knowledge to supply that localized information is unrealistic, so modernized short term data prediction strives to bring in additional sources of data to augment that vital localized knowledge and provide up-to-date insights in time to act on them.
Long-term demand prediction
Long-term demand prediction shifts the focus further out to look at what customer demand will look like in the next one to four years. This timeframe is generally appropriate for major business decisions such as expansion, diversification and new market entry. As with short-term demand prediction, institutional knowledge and experience are heavily relied upon to proactively identify major future events that will create disruption and opportunity. This drawback is greatly magnified over the long-term due to employee turnover and increased uncertainty, resulting in unforeseen anomalies and unrecognized opportunities that these old systems don’t show you.
Modernized long-term demand prediction relies on aggregated data from numerous internal and external sources to augment the knowledge of decision makers, resulting in a more complete picture of potential outcomes. As an example, few predicted the rapid move toward marijuana legalization across much of the United States due to its unprecedented nature. Companies in supporting industries with more extensive, data-driven long-term demand prediction were better able to see the change that was coming and position themselves to take advantage.
Internal capacity planning
Internal capacity planning is focused on ensuring that companies have the resources and staffing available to respond to customer demand. Can production lines keep up with increased orders? Is there enough warehouse space? Is there enough staff to run a production line 24/7 if needed? Traditionally the answers to those questions are based on the knowledge of senior staff and leadership. Consider flu season, which typically varies in severity from year to year. In years with more cases, drug stores can experience stock outages due to ineffective capacity planning by manufacturers, resulting in lost revenue. With modernized capacity planning, the full universe of data can be combined with machine learning to predict the severity of a flu season more accurately, enabling both the manufacturer and retailer to plan for the most likely scenarios.
Bringing it all together: Macroeconomic demand prediction
The keys to accurate demand prediction going forward lie in the volume of external data being consumed and the speed with which models can return accurate predictions. Modern demand prediction models fully utilize the human-powered institutional knowledge of experienced decision-makers and augment that valuable knowledge with external data from as many sources as possible. Information on economic conditions, consumer trends, geopolitical events and weather information should all influence demand prediction, as they influence consumer demand in the real world.
Even when fully modernized, these five methods of demand prediction will not work in isolation. Rather, they need to be used in conjunction to provide different lenses on future customer demand to provide the most realistic view on the future.
By better understanding demand, companies can price appropriately, purchase and store inventory more efficiently and reduce backorders – all of which makes for happier customers that won’t go looking to competitors. Getting demand forecasting right is a critical element of every company’s success, and Blueprint has the experience to improve any organization’s demand forecasting, as well as inventory and supply chain systems. Let’s have a conversation about your unique needs and how we can develop and implement the solutions that will help you satisfy your customers now and into the future.