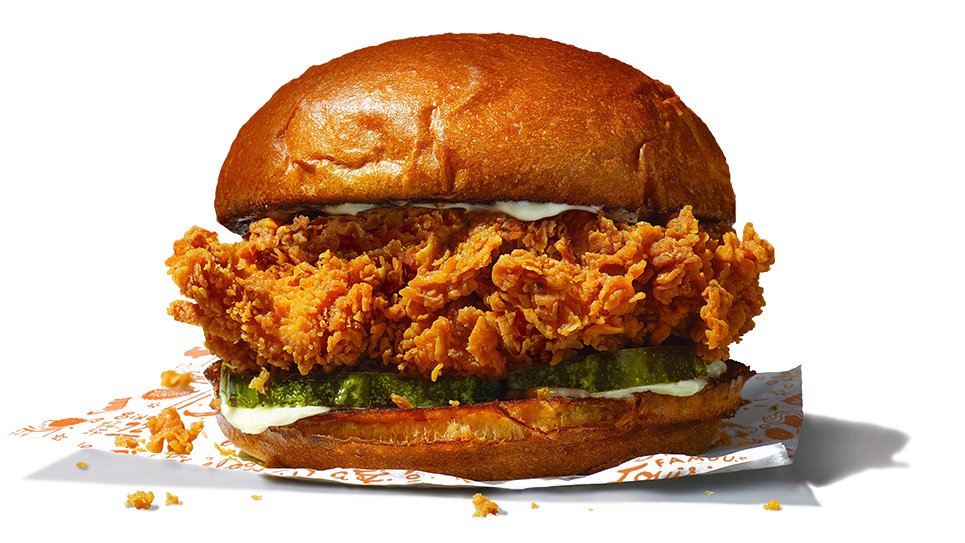
These days, all it takes is a trending hashtag for a company to see a huge influx in customers and therefore sales. Look at Popeyes and the #ChickenWars with Chick-fil-A that went viral, causing Popeyes to run out of chicken for a new sandwich in just two weeks. Or Ocean Spray and the #DreamsChallenge after a TikTok video of a man skateboarding while drinking the company’s cran-raspberry juice with Fleetwood Mac’s “Dreams” playing in the background went viral. To keep up with demand, Ocean Spray had to increase production and hire seasonal employees.
In the age of social media where Kylie and Kendall Jenner each have more than 150 million followers on Instagram, a single post from either can change the direction of a company in an instant.
As technology changes how customers shop, dine and even commute, establishing a modern inventory management system is the most critical thing restaurant and retail companies need to remain competitive.
U.S. Grocery stores operate on margins of 1% or 2% and restaurants run between 3% and 6% depending on the type of restaurant. Other retail can range from 2% to almost 8% based on sub-sector. Traditional inventory management solutions are no longer up to the task when a new celebrity collaboration can change everything overnight – like McDonald’s collaboration with rapper Travis Scott that caused the company to run out of ingredients for the Quarter Pounder.
While virality is widely unpredictable, for restaurants and retail, everything from weather and local sporting events to real-time traffic can cause disruptive inventory swings. Planning for and predicting these events that impact sales requires a nimble infrastructure, so the company knows what’s happening immediately and can react in the moment when feasible – there’s only so much chicken supply one can instantly create.
To increase margins, restaurants and retailers should do the following:
- Incorporate as much data as possible, so they can react in real time
- Have the flexibility to add new data as it becomes available
- Let that data work for them, allowing them to predict trends, trust the process and reduce dependency on humans
All the data you can eat
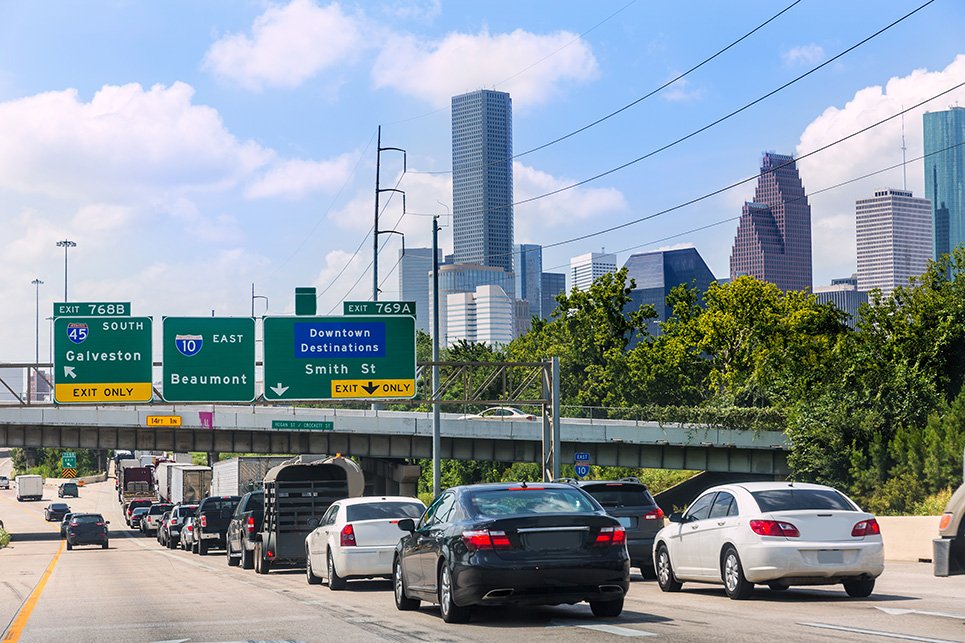
As one of the top-10 worst cities for traffic in the country, Houston, Texas, is also the number one city in the country for fast food consumption with more fast-food restaurants than any other city in the country. Coincidence? Absolutely not. When someone’s commute is expected to be 45 minutes and turns into 2 hours, guess what? That chicken cordon bleu they were so excited to cook themselves turns into a Quarter Pounder from McDonald’s on the way home.
It’s not uncommon for companies to make decisions based on 6-week-old data because they don’t have the infrastructure for real-time data injection or analysis. But if McDonald’s pulls all available data on scheduled road maintenance and/or live accident data and combines that with data about home games for the Astros and any conventions going on in the city into their data estate, they can create live dashboards for managers and inventory needs can be better predicted for specific stores based on weekly or daily activities.
The first step to managing and predicting inventory is establishing a modern data estate (MDE) that makes data consumption as broad and easy as possible. An MDE allows you to not only have all your internal data in one place and accessible, but it integrates external data like trends, demographics, weather, traffic, hotel and hospital stays – all data that a traditional data warehouse and BI system could not easily handle. Funneling all this data into real-time dashboards and BI platforms allows you to not only predict inventory needs in advance but gives a company the agility to manage and react to fast-developing situations. The inventory manager doesn’t need to wait for marketing to tell him they saw something trending on social media. He sees it happening in real time because social analytics and sales data are both piped into the modern data estate that feeds BI dashboards. He sees the spike as it is happening and can pivot in real time.
As the company grows and even more data becomes available, a modern cloud-based infrastructure can scale along with a company, giving them the flexibility to constantly add new data as it becomes available without the heavy lifting that comes with more traditional methods of data ingestion.
Let the data do the work
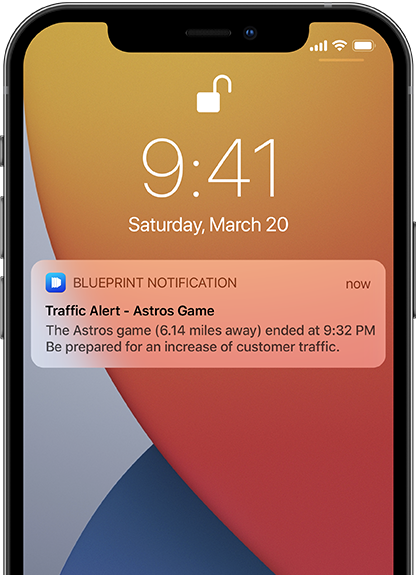
Companies that are simply comparing data year over year by day as a basis for inventory demand and predictions are stuck in the past. Imagine if restaurants in Seattle’s Pioneer Square are making inventory predictions solely based on dates – they’d completely miss that the Seattle Sounders FC home opener in 2021 is on April 16, not March 1 like it was in 2020. This event draws thousands of people to Pioneer Square, and in turn all the restaurants and bars in the area, for the March to the Match at the stadium, and to watch the match at nearby sports bars.
Modern inventory management is about bringing in all possible data so companies can react in real time and adapt their inventory quickly and smartly. Some restaurants prefer not to deliver because fries get mushy when not served fresh, so imagine how critical weather and traffic data become because both can deter consumers from picking up their own orders. Predictability can be expanded even further, though, by incorporating machine learning into a modern data estate, taking the strain off the shoulders of analysts or store managers and to more quickly understand what is happening in a given situation.
When a company simply compares one store or restaurant to another in its region, it rarely provides valuable insight for a company since factors can vary widely from location to location. This is where cohorts should be established. For example, Chili’s does not benefit from comparing sales at one Dallas suburb to another. Instead, using advanced analytics, store cohorts can be created based on factors such as foot traffic, local demographics, surrounding retail, populations and any other factor that they want to incorporate. Then those stores can be compared, allowing executives to make more relevant, informed decisions when comparing store-to-store metrics.
Traditionally, a company’s inventory management is only as good as a restaurant or store manager – inventory counts and decisions rely on the calculations and assumptions of that person. That is a situation that could easily be improved with the use of machine learning, not necessarily to replace the manager’s decision making, but to supplement it.
In one instance, Blueprint used machine learning to take the responsibility of inventory out of the manager’s hands and moved it to the supply chain team at the corporate level. Blueprint took a slice of historical inventory and sales data from a quarter of their thousands of stores nationwide, incorporated data on outside factors like the ones discussed in this piece, creating models to analyze trends, and within 6 weeks the algorithms exceeded the average inventory accuracy to the tune of a $15M/year ROI. That algorithm’s accuracy continues to improve over time as it learns and is refined by the data scientists behind it and as more external data is added. Read how that engagement started here.
Machine learning should be looked at as a cost optimization tool. Companies can avoid losses on unused inventory, key for retail and restaurants that are dealing with so many perishables, while also meeting real-time demands for increased staffing and inventory. Machine learning is necessary to optimize everything from advertising and pricing to marketing and supply chain management. To achieve any of these goals, it is imperative to incorporate as much external data as possible that impacts a company’s specific industry – with restaurants and retail that includes niche market from quick service to fine dining to grocery to auto parts.
Let’s have a conversation about how your data can do more to help your business, and how you can set up the best data estate to make that data work for you.