The data analytics journey
At Blueprint, our quest for innovation in Data and AI technologies has led us to a thrilling development this year—the establishment of our LLM Center of Excellence. This venture involves crafting tailor-made solutions for diverse industries, including manufacturing, retail, financial services, and health & life sciences.
We’ve long relied on the data analytics maturity model, a roadmap guiding us from explaining past events with structured data to predicting future occurrences and ultimately informing strategic decisions. However, navigating through these stages involves intricacies, combining data engineering, data shaping, platforms, machine learning algorithms, and data science techniques.
Descriptive
- Past & Current
- BI & Reporting
- Structured Data
Predictive
- Future
- Probabilities
- Similarity
Prescriptive
- Outcomes
- Actionable
- Measurable
Figure 1: Revised Information Maturity Model
Balancing the effort with the outcome
While the traditional model is robust, there are challenges along the way—points where the effort invested may outweigh the benefits. Shaping data into a useful form for insightful analysis can be tricky. Furthermore, not everyone is fluent in SQL or Python, leaving analysts dependent on pre-set models within BI tools.
This is where Large Language Models (LLMs) come to the rescue, acting as a bridge between human language and technical languages like SQL and Python. They democratize data, enabling individuals less inclined towards technical aspects to perform complex data analysis. Take a look at Figure 2 for a glimpse of Databricks’ LakehouseIQ, championing a new approach to data analysis using plain English as the new programming language.
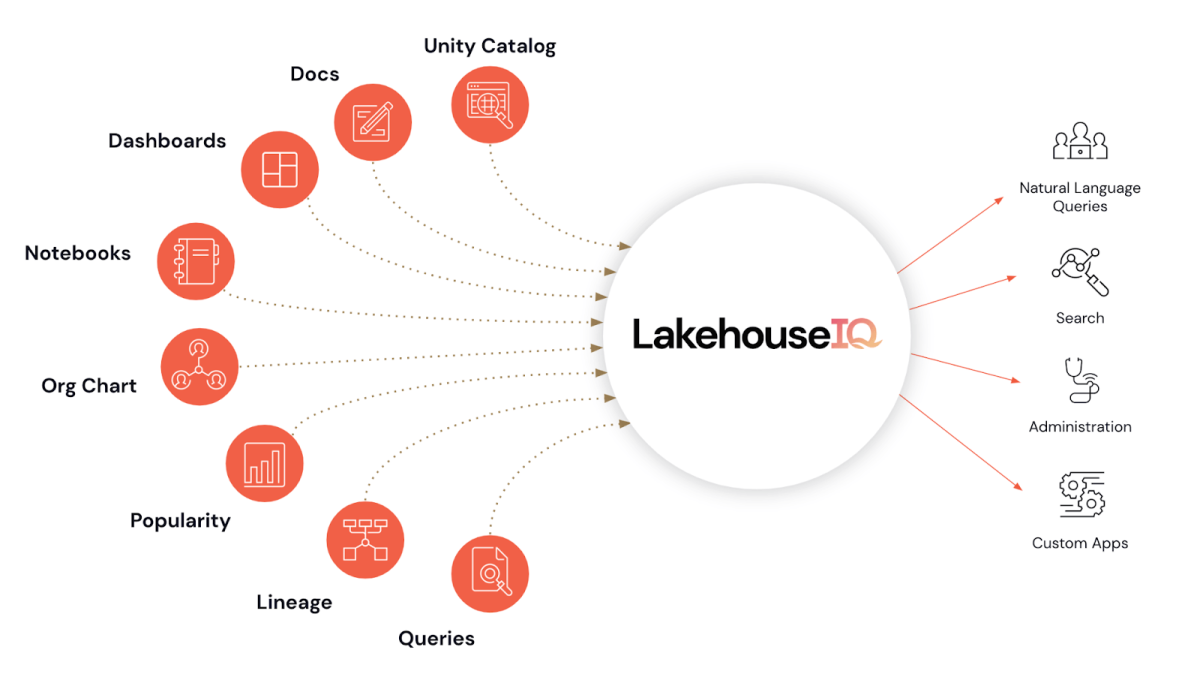
Figure 2: Databricks’ LakehouseIQ
Databricks continuously pushes the boundaries of what LLMs can achieve, offering features like sentiment analysis and text classification without burdening IT or data warehouse teams. LLMs provide predictive insights, generating queries to explore trends, outliers, and other intriguing data facets.
The potential applications of LLMs are vast, and we are eager to help your enterprise explore these language models’ capabilities to make data more accessible.
Making Sense of unstructured, structured, and media data
The traditional maturity model often overlooks insights hidden within unstructured data such as text, emails, content, videos, and images. According to the MIT Sloan School of Management, 80-90% of all data comprises unstructured information.
To unlock your organization’s data potential, a shift in the maturity model is imperative in the era of GenAI and LLM-powered applications.
Towards a new model
Having traversed the traditional analytics maturity model, we now recognize the need for a new model in the era of GenAI applications. Let’s explore how LLMs can be applied step by step, bringing increasing value to help enterprises tackle complexity and risk.
Information Maturity Model
Descriptive
pREDICTIVE
PRESCRIPTIVE
LLMs for
Understanding
The first phase of our LLM journey involves harnessing their exceptional language understanding abilities. Both commercial and open-source LLMs can aid in creating content, translation, QA, summarization, understanding context, and extracting entities.
This step doesn’t require complex tweaking; tools like “CoPilot” are already in use in Microsoft Office and Google Suite. We encourage businesses to extend LLM use beyond collaboration suites to analyze data from various sources such as chat history, customer tickets, client notes, contracts, and compliance documents.
LLMs for
Reasoning
The second leg of our journey sees LLMs evolving from language understanding to reasoning based on context, allowing for more complex, predictive outputs.
In a business setting, LLMs can be employed for:
Compliance
In industries like finance or healthcare where rules are always changing, LLMs can be trained on regulations and help evaluate company documents for compliance issues.
Staff training
LLMs can be fed company procedures, standards, and protocols, and then used to answer queries from employees during training.
Risk assessment
In the insurance industry, an LLM can be fed a client’s profile and reason through possible risk scenarios.
Multimodal reasoning
Integrating visual and textual data could give LLMs a richer understanding of situations, helping them predict outcomes and provide insights based on a wider range of input data.
LLMs in
Action
Our final destination is reached when LLMs can autonomously execute actions, building trust in their ability to understand and reason. Coupled with Robotic Process Automation (RPA) tools and APIs, LLMs become the ‘brain,’ interpreting inputs and making decisions, while RPAs act as the ‘hands,’ implementing decisions across systems.
Applications include supply chain management, customer service automation, and workflow automation. However, introducing LLMs into critical business processes requires careful consideration of factors like model accuracy, accountability, ethics, and security.
The journey ahead
It’s not a matter of if businesses will adopt LLMs, but when. As companies embrace AI, LLMs will play an increasingly prominent role in driving efficiency and value. At Blueprint, we’re committed to guiding our clients through this journey—from understanding to reasoning and finally to action.
Tech adoption should proceed step-by-step, aligning with organizational goals. While embarking on this transformative journey, our focus remains on ensuring model robustness, data privacy, governance standards, and top-notch security. With Blueprint as your guide, the journey with LLMs promises to be secure, efficient, and value-driven.
LLM Center of Excellence